Beyond the Forecast: The Tech Powering Next-Gen Climate Models
Paris, France | Jan 31, 2025
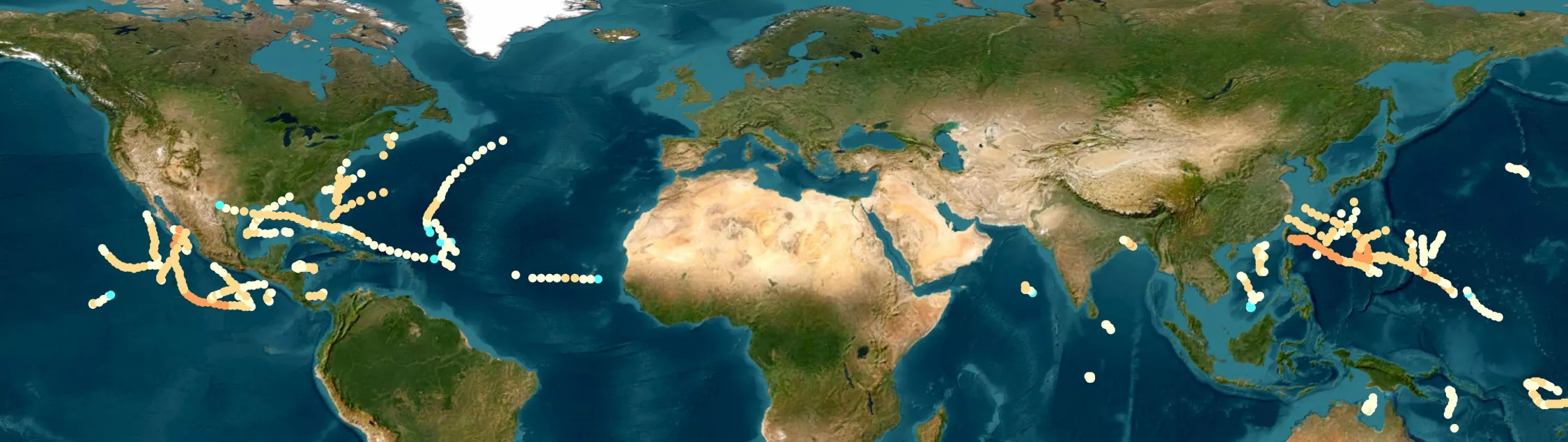
Sharon Howe, VP, Business Support with Viridien’s HPC & Cloud Solutions team recently spoke to Nicholas Heavens, Innovation Project Manager with Viridien’s Climate Modeling group, about how businesses can harness the power of HPC and AI to mitigate climate risks.
Nick has a degree in geophysical sciences from the University of Chicago, a PhD in planetary science from Caltech. He has also won numerous research grants from NASA and the National Science Foundation and published more than 65 peer-reviewed papers. At Viridien, he leads climate modeling innovation as part of the Ventures group.
Sharon has over 25 years of experience in the energy industry, having held various technical positions in subsurface imaging and integrated geoscience. Today, she focuses on helping clients from a wide range of compute-intensive industries to drive business innovation with Viridien’s advanced HPC, AI and cloud solutions.
From awareness to action: the evolution of climate science
Sharon: Climate change is a pressing global issue. How have you seen climate science evolve?
Nick: Climate science has evolved from helping raise awareness of human-driven climate change to identifying and managing risks, such as property damage from flooding or storms. Businesses, particularly in industries like insurance, must now disclose climate risks, much like they do for cyberattacks or interest rate changes.
However, current climate models are limited. Low-resolution models treat extensive regions like Tokyo or Los Angeles as single points. Yet, anyone that lives there will tell you the weather can vary widely across these sprawling areas. Higher resolution is the goal, but it can come at significant cost—a 10x improvement in resolution in the models used to predict weather over Europe requires 1,000x more computing power, and climate forecasts need to span years, not just days.
To address the limitations of low-resolution climate models, researchers use techniques like downscaling. This approach integrates historical data into forecasts to enhance detail, making it possible to correct model flaws or blend strengths from multiple models. While downscaling offers an accessible solution for working on your desktop or with a small cluster, balancing accuracy and affordability remains a key challenge.
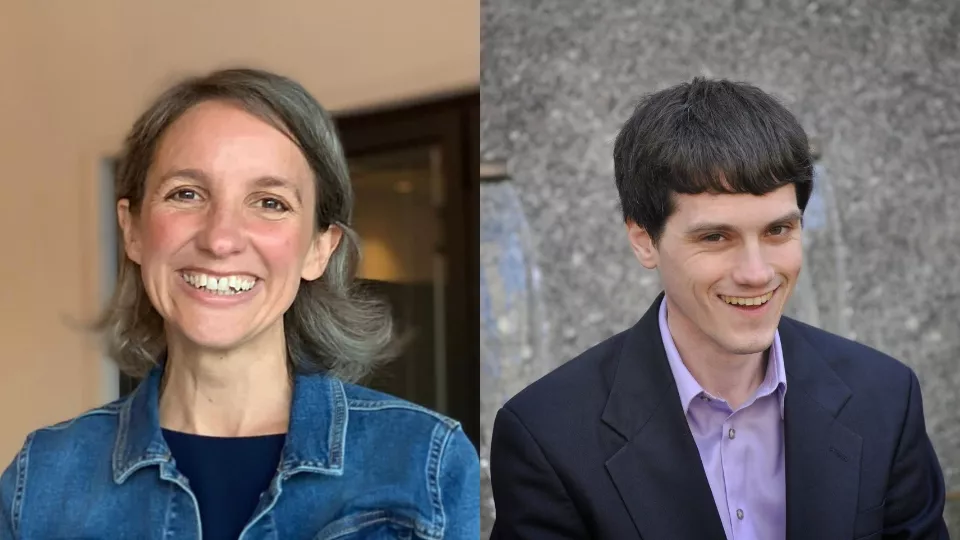
Why HPC is critical for today’s climate forecasting
Sharon: Companies need climate forecasts to cover the lifetime of investments. Can models achieve that level of detail affordably?
Nick: We can't predict specific weather events far in advance, but we can identify trends. For example, while we can’t forecast the exact day a hurricane will hit Houston, we can estimate the likelihood of it happening over the next 30 years.
Climate models simulate the movement of fluids like air and water in three dimensions using complex equations like Navier-Stokes. These systems are divided into components—like atmosphere and ocean—and rely on HPC to manage the massive computations. For instance, running a 1-degree model can take 16 days on 720 Intel cores to simulate a century. If you wanted to scale to higher resolutions, you’re talking about a very long time or impractically large number of cores.
Interestingly, the motivation for early digital computers stemmed from weather forecasting. In the early 1920s, Louis Fry Richardson envisioned weather calculations being conducted like a symphony orchestra, with a bunch of people in a room using pencil and paper, and a conductor guiding them through the calculations—of course, very impractical! Really, HPC is Richardson’s orchestra, translated to a lot of electronic components.
Integrating models for actionable climate data
Sharon: What sets Viridien’s high-resolution climate modeling capabilities apart?
Nick: We combine widely used models for the atmosphere, ocean and waves with innovative techniques. For example, MPAS-A, a global weather model, lets us focus high-resolution grids on specific areas, such as Europe or North America, while leaving the rest of the globe at lower resolution. This approach saves up to 90% of the computational load.
We can then integrate popular European/American ocean and wave models for the other components and take advantage of the capabilities of each in specific ways to create realistic projections of future weather from low-resolution forecasts. This approach to combining insights is unique to Viridien so far.
Our goal is to provide actionable data to industries like insurance, real estate and energy—offering precision and client-focused solutions that public services often cannot deliver.
Maximizing efficiency with code and GPU optimization
Sharon: High resolution means more computation. How are we addressing that challenge?
Nick: We’re bridging the gap with two main techniques: code optimization and graphical processing unit (GPU) offloading.
Currently, code is built for diverse processor architectures, but it’s like knowing many words in a language without knowing the precise term—you end up rambling on about the long, green, crunchy watery plant you eat with your favorite dip instead of… celery! So, we need to make the code speak more efficiently to machines.
GPUs are another game-changer. They’re energy-efficient but can be challenging to scale. Fortunately, Viridien has decades of expertise in industrial-scale GPU optimization. Really, we were working with GPUs before there was a language to program them! This helps us explore new techniques to speed up computation and reduce costs.
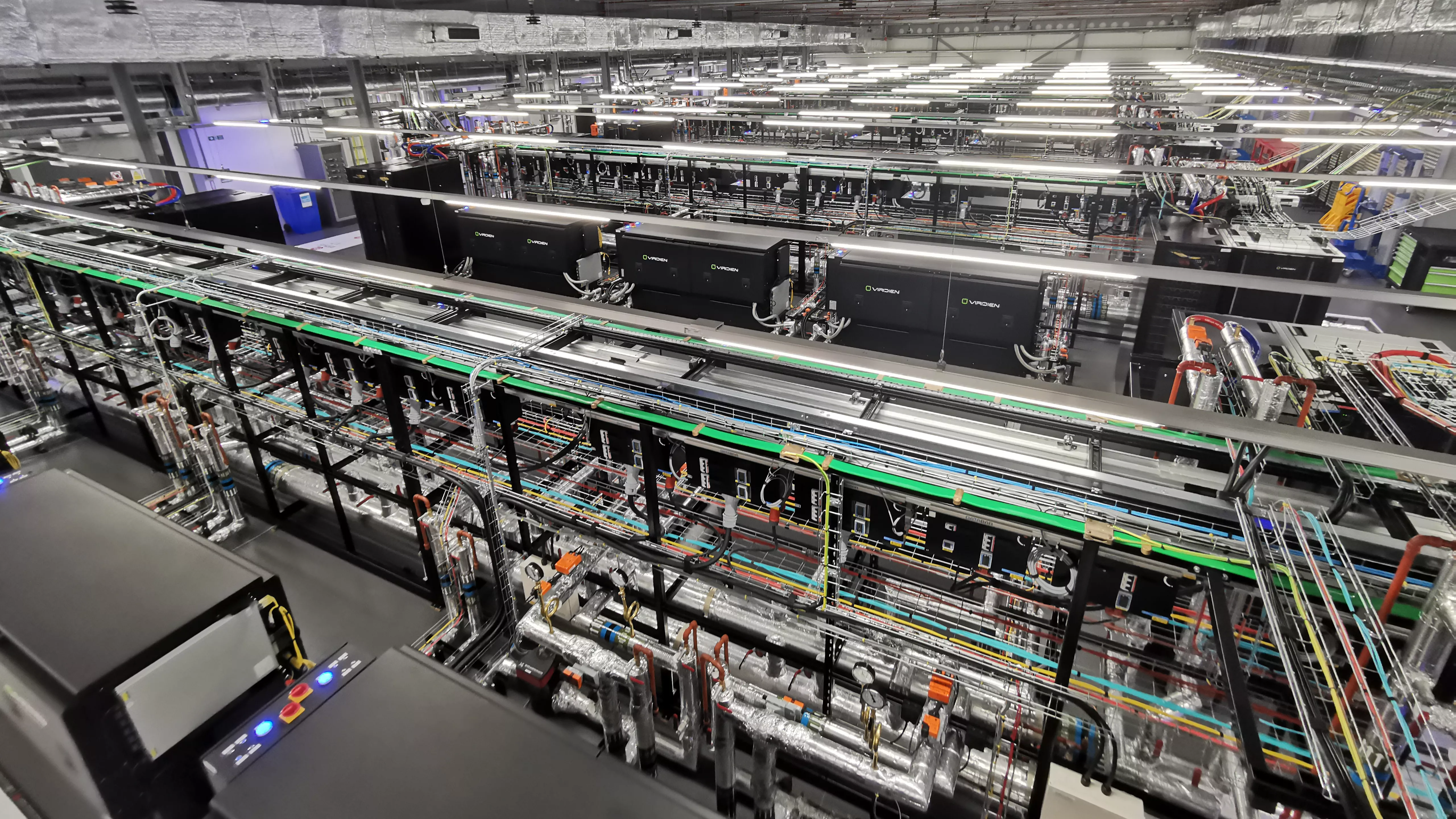
Looking ahead: machine learning and practical limits
Sharon: What does the future of climate modeling look like?
Nick: Models will likely reach practical resolution limits soon, somewhere between 1 and 10 km. For business applications, this may not be such a bad thing. Flood risks, for example, often depend more on local infrastructure than precise weather data. If a thunderstorm at 3 km away drops a lot of rain, you’re more likely to be flooded because of the drainage patterns around you than your proximity, so forecasts at the scale of an office building are overkill.
Machine learning (ML) is a promising tool for the future. While current efforts focus on downscaling and replicating weather models, ML-driven algorithms could eventually cut computational loads by up to a quarter. The future could lie with hybrid models that combine ML with traditional physics-based approaches.
Learn more about Viridien's HPC, AI & cloud solutions
Learn more about Viridien's Ventures group