Technical Abstract
Data-driven method for training data selection for deep learning
Back to Technical ContentConvolutional based deep neural networks can be used in addition to existing workflows, to improve turnaround or as a ‘guide’ for further processing. Whilst a lot of effort has been made to try to improve the DNN architecture for processing tasks or to understand their physical interpretation, the choice of the training-set is rarely discussed. For a good quality DNN result, the training-set must be representative of the variability (or statistical diversity) of the full dataset, and the question of the choice of this dataset for seismic data is discussed in this paper. We present two methods for the selection of the training set. The first one is based on proxy attributes and their clustering. Our clustering approach is not only using the clusters themselves but also the information on the distance to the centroid for the cluster definition. The other method is based on the data themselves. It starts from a predefined training set and then scans through the full dataset to identify additional training points that will be used to augment the initial training set.
Download Resource 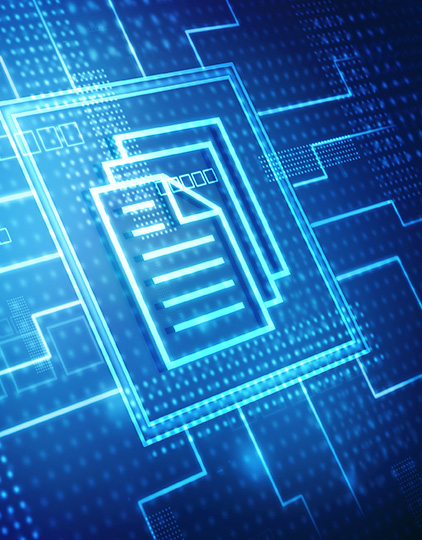
Publications
EAGE - European Association of Geoscientists and EngineersAuthors
Celine Lacombe, Issa Hammoud, Jeremie Messud, Hanyuan Peng, Thibault Lesieur, Paulien Jeunesse