Technical Abstract
Introducing Deep Neural Networks for Ultra-Fast Track Processing: A New Early-Out Product for Qc and Interpretation
Back to Technical ContentTo improve the imaging of the Barents Sea’s Nordkapp basin, either in terms of resolution or geological structure, a new seismic acquisition design was proposed, using a widespread hexa-source sitting on top of 18 non-flat streamers. Imaging this new recorded data required specific and complex processing steps using advanced algorithms to maintain high spatial resolution. This were not easy to handle onboard or through a fast-processing flow, so we implemented an Ultra-Fast Track processing by leveraging the capability of Deep Neural Networks to perform the pre-processing stages, ensuring quality in a limited timeframe. Pseudo-synthetic training sets were built from the first batch of received data and data augmentation was applied, with different setting for each processing steps. This result showed an improved resolution with respect to the legacy volume and was used to start interpreting potential hydrocarbon prospects and initiate the velocity model building. The Ultra-Fast Track also served as a helpful tool to assess the remaining challenges to be faced during the full processing.
Download Resource 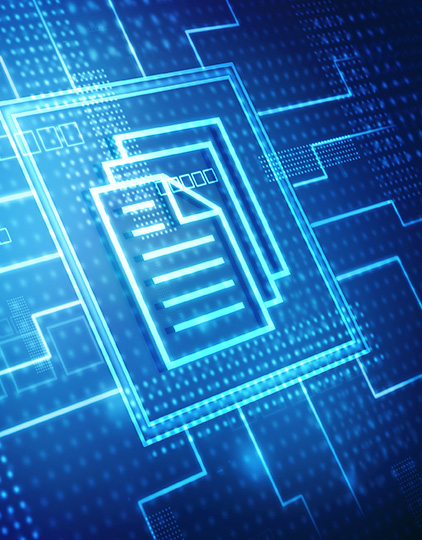
Publications
EAGE - European Association of Geoscientists and EngineersAuthors
Guillaume Gigou, Loic Janot, Nicolas Salaun, Sylvain Masclet, Isabel Espin (CGG) ; Per Eivind Dhelie, Vidar Danielsen, Jan Erik Lie (Lundin Energy)